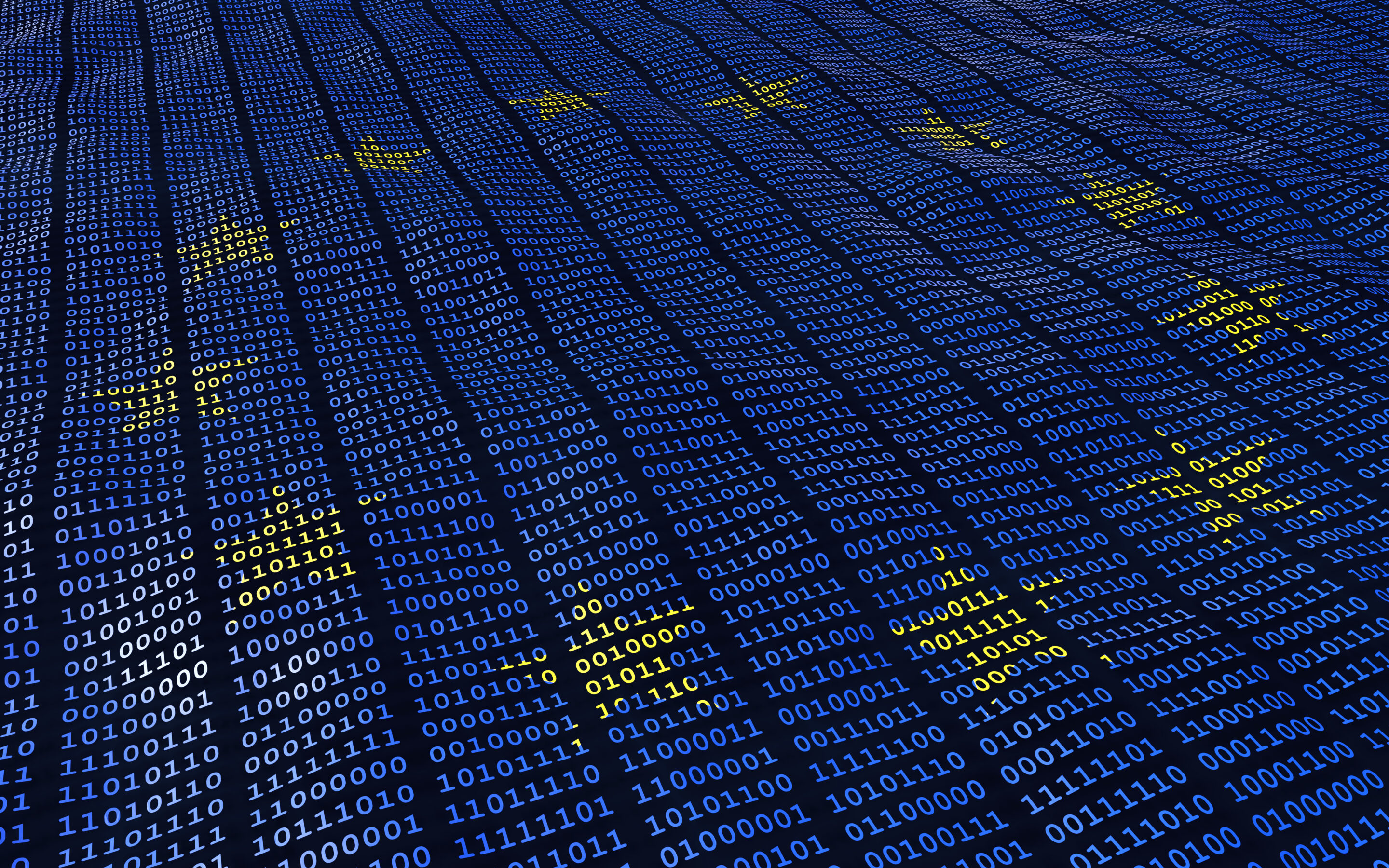
Our contribution in support of future European AI regulation
Confiance.ai produces technologies and methodologies that can be used to help companies meet the requirements of future regulations (AI Act).
A three-level approach
The European approach to trustworthy artificial intelligence can be analyzed as consisting of three levels.
Regulation is the top level: applicable in the long term, it sets out the requirements that AI systems, particularly those qualified as high-risk, must meet if they are to be deployed in the service of European citizens: transparency, traceability, robustness, precision, controllability, etc.
At the intermediate level, harmonized standards, the development of which has been entrusted to the CEN/CENELEC organization, will specify how the high-level requirements of the regulations will be translated into concrete requirements for AI systems that organizations will have to implement, and which will eventually be verified by third parties (“notified bodies”).
Harmonized standards will contain requirements for the design, development and supervision of systems, as well as for the AI system products themselves. The third level is therefore that of concrete implementation of the requirements, and in particular the tools and methods used to achieve them. This is where the Confiance.ai program comes in, with its focus on providing methods and tools to improve confidence in AI systems for mission-critical applications. The tools and methods produced by Confiance.ai are aimed primarily at mission-critical systems, but by extension can also be applied to other applications.
Technological contributions
Confiance.ai’s technological contributions to European regulations mainly concern three requirements, embodied in three of the ten requests for standards made to CEN/CENELEC by the European Commission:
- Robustness, i.e. the system’s ability to perform its intended function in the presence of abnormal or unknown inputs. Confiance.ai has produced and tested some twenty software components on this subject.
- Accuracy: a quantitative measure of the magnitude of the AI system’s output error. Around ten of the components tested and produced as part of Confiance.ai are relevant to improving system accuracy or precision.
- Data quality: the extent to which data is free from defects and possesses the desired characteristics for the intended application. On this subject too, Confiance.ai has produced and evaluated a dozen components as well as a specific platform bringing together various approaches to improving the quality of input data for machine learning systems.
These tools are referenced in the catalog produced by Confiance.ai, and several of them, in open source, are available for download.
Confiance.ai’s technological contributions don’t stop at these three properties: we have also made contributions to other regulatory requirements, translated into requests for harmonized European standards: on explainability (corresponding to the need for transparency and understanding of AI systems by humans); on the cybersecurity of AI systems, notably through the watermarking of AI system outputs – images or other content; and more generally on risk control and compliance analysis. Here too, all software components are listed in the online catalog, and some are available for download.
Methodological contributions
Confiance.ai’s methodological contributions cover the development process of an AI system, from initial specification and design to commissioning and supervision of its operation, including in embedded systems. These contributions are manifold:
- a taxonomy of concepts and terms used in trustworthy AI ;
- a complete documentation of the process, including modeling of activities and roles, with elements enabling corporate engineering departments to implement it;
- A first development of a Trustworthy AI ontology, linking the main concepts of the process and the taxonomy;
- And a “Body of Knowledge” which brings together all these elements and makes them accessible on the website of the same name.
While the use of these methodological tools is not in itself a guarantee of the AI system’s compliance with regulations, it can constitute an element of justification, considered to be part of the state of the art by the notified bodies in charge of verification.
Figure 1: Systems engineering and software engineering lifecycle
Direct contributions to standards
Finally, Confiance.ai was involved from the outset in the working groups set up to produce the harmonized European standards presented above as the second level of the approach. We thus
- provided input on the taxonomy of trustworthy AI and a vision of the set of attributes contributing to trust in an AI system;
- submitted an original contribution on the definition and use of the notion of operational design domain, which could be integrated into European and international standards;
- played an active role in the working group responsible for producing the European standard on AI risk management, notably by drafting a catalog of sources of risk and known responses in the literature;
- Finally, our support for various initiatives to label AI products and companies designing AI products is clearly linked to future regulations, since future labels will also be based on these regulations.
Confiance.ai’s industrial members are convinced of the need to improve trust in AI systems, particularly in the context of critical systems involving the health and safety of people and property. This conviction is in line with the objectives of future European regulations on AI; Confiance.ai is materializing this ambition by developing and evaluating a set of tools and methods, as well as participating in the ongoing process of establishing standards for this purpose.